تاريخ الرياضيات
الاعداد و نظريتها
تاريخ التحليل
تار يخ الجبر
الهندسة و التبلوجي
الرياضيات في الحضارات المختلفة
العربية
اليونانية
البابلية
الصينية
المايا
المصرية
الهندية
الرياضيات المتقطعة
المنطق
اسس الرياضيات
فلسفة الرياضيات
مواضيع عامة في المنطق
الجبر
الجبر الخطي
الجبر المجرد
الجبر البولياني
مواضيع عامة في الجبر
الضبابية
نظرية المجموعات
نظرية الزمر
نظرية الحلقات والحقول
نظرية الاعداد
نظرية الفئات
حساب المتجهات
المتتاليات-المتسلسلات
المصفوفات و نظريتها
المثلثات
الهندسة
الهندسة المستوية
الهندسة غير المستوية
مواضيع عامة في الهندسة
التفاضل و التكامل
المعادلات التفاضلية و التكاملية
معادلات تفاضلية
معادلات تكاملية
مواضيع عامة في المعادلات
التحليل
التحليل العددي
التحليل العقدي
التحليل الدالي
مواضيع عامة في التحليل
التحليل الحقيقي
التبلوجيا
نظرية الالعاب
الاحتمالات و الاحصاء
نظرية التحكم
بحوث العمليات
نظرية الكم
الشفرات
الرياضيات التطبيقية
نظريات ومبرهنات
علماء الرياضيات
500AD
500-1499
1000to1499
1500to1599
1600to1649
1650to1699
1700to1749
1750to1779
1780to1799
1800to1819
1820to1829
1830to1839
1840to1849
1850to1859
1860to1864
1865to1869
1870to1874
1875to1879
1880to1884
1885to1889
1890to1894
1895to1899
1900to1904
1905to1909
1910to1914
1915to1919
1920to1924
1925to1929
1930to1939
1940to the present
علماء الرياضيات
الرياضيات في العلوم الاخرى
بحوث و اطاريح جامعية
هل تعلم
طرائق التدريس
الرياضيات العامة
نظرية البيان
FUZZY SETS-Expanding Concepts of Fuzzy Set
المؤلف:
Kwang H. Lee
المصدر:
First Course on Fuzzy Theory and Applications
الجزء والصفحة:
14-20
5-7-2016
1978
Expanding Concepts of Fuzzy Set
Example of Fuzzy Set
Example 1.1 Consider a universal set X which is defined on the age domain.
X= {5, 15, 25, 35, 45, 55, 65, 75, 85}
Table 1.1. Example of fuzzy set
We can define fuzzy sets such as “infant”, “young”, “adult” and “senior” in X. The possibilities of each element ofx to be in those four
fuzzy sets are in Table 1.1
We can think of a set that is made up of elements contained in A. This set is called “support” of A.
The support of fuzzy set “young” is,
Fig. 1.1. Non-Normalized Fuzzy Set and Normalized Fuzzy Set
Support(youth) = {15, 25, 35, 45, 55}
and it is a crisp set. Certainly, the support of “infant” is empty set.
The maximum value of the membership is called “height”. Suppose this “height” of some fuzzy sets is 1, this fuzzy set is “normalized”. The sets
“young”, “adult” and “senior” are normalized (Fig 1.1).
Let’s consider crisp set “teenager”. This crisp set is clearly defined having elements only 10-19 in the universal set X. As you shall notice this
set is a restricted set comparing with X. Similarly fuzzy set “young” is also a restricted set. When we apply a “fuzzy restriction” to universal set X in
certain manner, we get a fuzzy set
1.2 α -Cut Set
Definition ( α -cut set) The ⍺-cut set A⍺ is made up of members whose membership is not less than ⍺.
note that ⍺ is arbitrary. This ⍺-cut set is a crisp set (Fig 1.2).
Example 1.2 The α -cut set is derived from fuzzy set “young” by giving 0.2 to α
this means “the age that we can say youth with possibility not less than 0.2”.
When two cut sets A α and A⍺’ exist and if α ≤ α ' d for them, then
the relation Young0.2 ⊇ Young0.8, for example, holds.
Fig. 1.2. α -cut set
Definition (Level set) The value D which explicitly shows the value of the membership function, is in the range of [0,1]. The “level set” is obtained
by the α ’s. That is,
The level set of the above fuzzy set “young” is,
1.3 Convex Fuzzy Set
Definition (Convex fuzzy set) Assuming universal set X is defined in n-dimensional Euclidean Vector space Rn
If all the ⍺- cut sets are convex, the fuzzy set with these α - cut sets is convex(Fig 1.3). In other words, if a
holds, the fuzzy set A is convex.
Fig 1.4 shows a convex fuzzy set and Fig 1.5 describes a non-convex set.
Fig. 1.3. Convex Fuzzy Set
Fig. 1.4. Convex Fuzzy Set
Fig. 1.5. Non-Convex Fuzzy Set
1.4 Fuzzy Number
“Real number” implies a set containing whole real numbers and “Positive number” implies a set holding numbers excluding negative numbers.
“Positive number less than equal to 10 (including 0)” suggests us a set having numbers from 0 to 10. So
A = “positive number less than equal to 10 (including 0)” = {x | 0 ≤ x ≤ 10, x ∊R }
since the crisp boundary is involved, the outcome of membership function is 1 or 0.
Definition (Fuzzy number) If a fuzzy set is convex and normalized, and its membership function is defined in R and piecewise continuous, it is
called as “fuzzy number”. So fuzzy number (fuzzy set) represents a real number interval whose boundary is fuzzy(Fig 1.6).
1.5 The Magnitude of Fuzzy Set
In order to show the magnitude of fuzzy set, there are three ways of measuring the cardinality of fuzzy set. First, we can derive magnitude by
summing up the membership degrees. It is “scalar cardinality”.
Fig. 1.6. Sets denoting intervals and fuzzy numbers
Following this method, the magnitude of fuzzy set “senior” (in the previous example) is,
|senior| = 0.1 + 0.2 + 0.6 + 1 + 1 = 2.9
Second, comparing the magnitude of fuzzy set A with that of universal set X can be an idea.
This is called “relative cardinality”. In the case of “senior”,
Third method expresses the cardinality as fuzzy set.
Definition (Fuzzy cardinality) Let’s try to get ⍺-cut set (crisp set) A α , of A. The number of elements is |A α |. In other words, the possibility for
number of elements in A to be|A α | is ⍺. Then the membership degree of fuzzy cardinality |A| is defined as,
where A α is a ⍺-cut set and ⩘ A is a level set.
Example 1.3 If we cut fuzzy set “senior” at α =0.1, there are 5 elements in the α -cut set. senior0.1 = {45, 55, 65, 75, 85}, |senior0.1| = 5. In the same
manner, there are 4 elements at α =0.2, there are 3 at α =0.6, there are 2 at α =1. Therefore the fuzzy cardinality of “senior” is
|senior| = {(5, 0.1), (4, 0.2), (3, 0.6), (2,1)}.
1.6 Subset of fuzzy set
Suppose there are two fuzzy setsA andB. When their degrees of membership are same, we say “A and B are equivalent”. That is,
If μ A(x) ≠ μ B(x) for any element, then A ≠ B. If the following relation is satisfied in the fuzzy set A and B, A is a subset of B(Fig 1.6).
This relation is expressed as A ⊆ B. We call that A is a subset of B. In addition, if the next relation holds, A is a proper subset of B.
This relation can be written as
Fig. 1.6. Subset A ⊂ B
Kwang H. Lee, First Course on Fuzzy Theory and Applications, 2005, Springer, pag(14-20)
الاكثر قراءة في الضبابية
اخر الاخبار
اخبار العتبة العباسية المقدسة
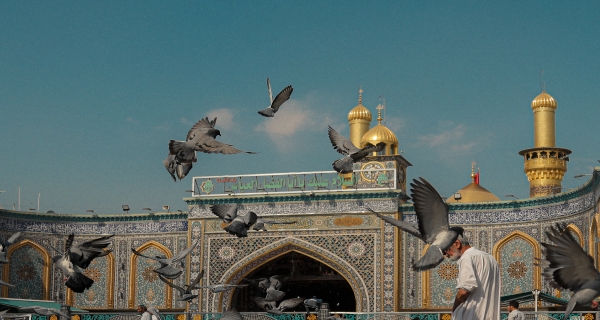
الآخبار الصحية
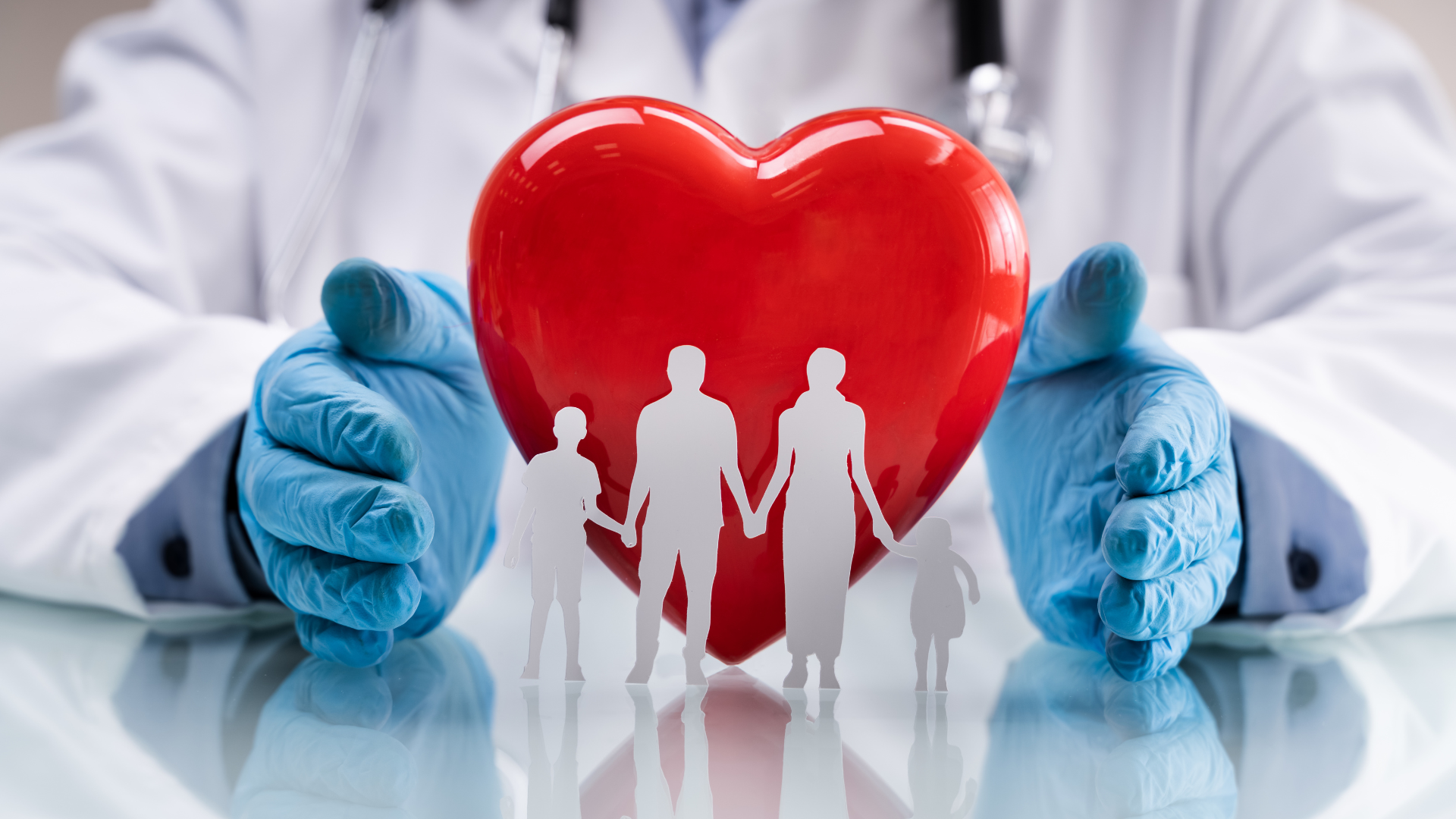