تاريخ الرياضيات
الاعداد و نظريتها
تاريخ التحليل
تار يخ الجبر
الهندسة و التبلوجي
الرياضيات في الحضارات المختلفة
العربية
اليونانية
البابلية
الصينية
المايا
المصرية
الهندية
الرياضيات المتقطعة
المنطق
اسس الرياضيات
فلسفة الرياضيات
مواضيع عامة في المنطق
الجبر
الجبر الخطي
الجبر المجرد
الجبر البولياني
مواضيع عامة في الجبر
الضبابية
نظرية المجموعات
نظرية الزمر
نظرية الحلقات والحقول
نظرية الاعداد
نظرية الفئات
حساب المتجهات
المتتاليات-المتسلسلات
المصفوفات و نظريتها
المثلثات
الهندسة
الهندسة المستوية
الهندسة غير المستوية
مواضيع عامة في الهندسة
التفاضل و التكامل
المعادلات التفاضلية و التكاملية
معادلات تفاضلية
معادلات تكاملية
مواضيع عامة في المعادلات
التحليل
التحليل العددي
التحليل العقدي
التحليل الدالي
مواضيع عامة في التحليل
التحليل الحقيقي
التبلوجيا
نظرية الالعاب
الاحتمالات و الاحصاء
نظرية التحكم
بحوث العمليات
نظرية الكم
الشفرات
الرياضيات التطبيقية
نظريات ومبرهنات
علماء الرياضيات
500AD
500-1499
1000to1499
1500to1599
1600to1649
1650to1699
1700to1749
1750to1779
1780to1799
1800to1819
1820to1829
1830to1839
1840to1849
1850to1859
1860to1864
1865to1869
1870to1874
1875to1879
1880to1884
1885to1889
1890to1894
1895to1899
1900to1904
1905to1909
1910to1914
1915to1919
1920to1924
1925to1929
1930to1939
1940to the present
علماء الرياضيات
الرياضيات في العلوم الاخرى
بحوث و اطاريح جامعية
هل تعلم
طرائق التدريس
الرياضيات العامة
نظرية البيان
Chaos
المؤلف:
Devaney, Robert L
المصدر:
A First Course in Chaotic Dynamical Systems
الجزء والصفحة:
...
5-1-2016
742
The word “chaos” is used in mathematics to mean something other than what it means in everyday English. In English, we speak of chaos as a state in which everything has gone awry, there is complete disorder, and there are no rules governing this behavior. In mathematics, chaotic systems are well defined and follow strict mathematical rules. Although chaotic systems are unpredictable, they do have certain patterns and structure, and they can be mathematically modeled, often by an equation or a system of equations.
Chaos theory is the study of systems that change over time and are inherently unpredictable. Some systems, such as Earth’s orbit about the Sun, are very predictable over long periods of time. Other systems, such as the weather, are notoriously unpredictable. Chaos theory builds a mathematical framework to account for unpredictable systems, like the weather, which behave in a seemingly random fashion.
The last two decades of the twentieth century saw tremendous advances in our understanding of chaotic systems. Chaos theory has been applied to the study of fibrillation in human hearts, the control of epileptic seizures, the analysis of how the brain processes information, the dynamics of weather, fluctuations in the stock market, the formation of galaxies, the flow of traffic during rush hour, and the stability of structures during earthquakes, to name a few. Wherever there are unstable or unpredictable systems, chaos scientists can be found attempting to reveal the underlying order in the chaos.
Early History of Chaos Theory
Although chaos theory is very much a late-twentieth-century development, most chaos scientists consider the great nineteenth-century mathematician, Henri Poincaré, to be the true father of the discipline. The fundamental phenomenon common to all chaotic systems is sensitivity to initial conditions, and Poincaré was the first to articulate this sensitivity in his study of the so-called three-body problem.
Poincaré had described this problem in 1890 when he attempted to calculate the orbits for three interacting celestial bodies. Poincaré found that Newton’s equations for two celestial objects were useless after a short time.
The orbits became so tangled that he gave up hope of trying to predict where they would go. No one quite knew what to do about Poincaré’s problem and so it remained essentially a mystery for the next eight decades.
Henri Poincaré is known for his statement of the principle of “sensitivity toinitial conditions,” which
says that small differences in the initial conditions lead to very greatones in the final phenomena,
so that predictionbecomes impossible.
Chaos in the Twentieth Century
In the 1950s meteorologists had great hopes that accurate weather prediction was about to become a reality. The advent of computers that could analyze vast amounts of data was seen as a great breakthrough in weather forecasting. Unfortunately, by the end of the decade, the optimism had begun to fade. Even with the rapid analysis of data from weather stations all over the world, forecasting did not seem to improve.
Meteorologist and mathematician Ed Lorenz of the Massachusetts Institute of Technology wondered why and began attempting to model the weather with equations programmed into his computer. The computer used the equations to simulate several months of weather, producing a graph that would rise and fall according to changes in the variables of Lorenz’s weather equations.
At one point Lorenz wanted to take a closer look at a certain part of his graph, so he went back to an earlier point on the plot, inserted the values for that point from a printout from the computer, and waited to see a repetition of the graph he had seen before. At first he did, but after a few minutes he was startled to see a new plot diverging from the old plot, creating an entirely different version of the weather. After checking his computer for a malfunction and finding none, he discovered the cause of this unexpected behavior.
When he had restarted the simulation, he had entered values from the printout that were rounded off to three decimal places, whereas the computer was using six place decimals for the simulation. This small variation in the initial values had produced a completely different version of the simulated weather.
By accident, Lorenz had rediscovered Poincaré’s sensitivity to initial conditions. He realized that this meant that unless one has infinitely precise knowledge of all the initial conditions in a weather system, one cannot accurately predict the weather very far into the future. Lorenz called this finding “The Butterfly Effect” because his results implied that the flapping of a butterfly’s wings in Brazil could stir up weather patterns that might
ultimately result in a tornado in Texas.
Fish Population Studies. In 1970, the biologist Robert May was studying the growth of fish populations using a well-known mathematical model for populations with an upper bound to growth. This upper bound is sometimes called the “carrying capacity” of the environment. The model was the logistic difference equation P(t + 1) = rP(t)(1 P(t)), where r is a number greater than 1 representing the growth rate, P(t) is the population as a
percentage of carrying capacity at time t, and the factor (1 P(t)) represents the fact that as P(t) gets closer to 1 (closer to 100 percent of the carrying capacity), the growth rate of the population slows to almost zero.
May had noticed that fish populations sometimes maintained the same population from one year to the next and sometimes would fluctuate from year to year. He decided to study what happened with the logistic equation when he varied the values of the parameter r, which essentially represent the growth rates of the population. The logistic difference equation is an iterator. This means that one inputs a certain initial population called P(0) into the equation, does the calculation, and receives as the output P(1), which is the population at the next time interval. Then this output value P(1) becomes the new input into the equation, yielding a new output P(2), and so on.
Using a simple hand calculator, May carried out hundreds of iterations (repetitions) using different values for the initial population, P(0), and different values of the growth parameter r. He discovered that when the value of r was between 1 and 3, the iteration produced a string of numbers that would ultimately settle on a single value no matter what initial value was used. This implied a stable population year after year. When r was raised
slightly above 3, however, the output of the iteration cycled back and forth between two values.
This remained true for all initial values until May raised the parameter beyond 3.45 and observed that the values began oscillating among four values, again independent of the initial population. At about 3.54, the oscillation doubled again to eight distinct values, then to sixteen when r was increased passed 3.556, then to thirty-two and sixty-four, and so on. When the parameter value reached about 3.56994, this “period-doubling cascade,” as May would call it, ended and the values seemed to jump about randomly.
At this point May noticed that the values produced by an iteration for a given r value were no longer independent of the initial value. For r = 3.6, for example, if the initial value were 0.1, the twentieth iteration was about 0.7977, but if the initial value were 0.09, the twentieth iteration was about 0.8635. The discrepancy became greater with additional iterations. Once again Poincaré’s sensitivity to initial conditions had been rediscovered. In
this case, May had discovered chaos in the logistic difference equation.
Feigenbaum’s Constant.
May’s period-doubling cascade became the subject of intense study by the physicist Mitchell Feigenbaum in the mid-1970s.
Whereas May had concerned himself with the qualitative aspects of the logistic system, Feigenbaum wanted to understand the quantitative basis of this period-doubling route to chaos, as it would come to be called. Starting with a handheld calculator and eventually making use of more powerful computers, Feigenbaum discovered a completely unexpected pattern associated with the parameter (r) values at which each new period doubling occurred.
He called these bifurcation values.
Taking the computations far beyond where May had taken them, Feigenbaum came up with exceptionally high accuracy for the bifurcation values. For example, the first six are b1 =3, b2= 3.449490. . ., b3 =3.544090. . ., b4= 3.556441. . ., b5 = 3.568759. . ., and b6 = 3.569692. . ..
So, for example, when the parameter is raised past b5 = 3.568759, the logistic equation’s output changes from an oscillation among sixteen values to an oscillation among thirty-two values. Feigenbaum had the computer draw a graph showing parameter values on the horizontal axis and population values on the vertical axis. The result, known as the Feigenbaum plot, is now one of the icons of chaos theory.
The Feigenbaum plot shows how the bifurcations come increasingly closer as the parameter is increased from left to right. When the parameter value passes 3.56994. . ., the period doublings are no longer regular. This is the onset of chaos.
As fascinating as this was, Feigenbaum found something even more amazing in these numbers. He found that if he calculated ratios of the form (bk+1 bk)/(bk bk1) for larger and larger values of k, these ratios would approach the number 4.669201609. . .. Furthermore, he found that if he used other functions, such as sine or cosine, completely unrelated to the logistic equation, the result would be the same. The number 4.669201609. . .
is now known as Feigenbaum’s constant and is considered to be one of the fundamental constants of mathematics.
The Link to Fractals.
The Feigenbaum plot exhibits self-similarity, meaning that any one of the branches looks like the entire plot. Figures that exhibit self-similarity are called “fractals,” a term invented by Benoit Mandelbrot in 1975. Just as Euclidean geometry is the geometry of Newtonian mechanics, fractal geometry is the geometry of chaos theory.
Geometrically, the dynamics of chaotic systems are described by figures called “attractors,” of which the Feigenbaum plot is one example. Although these dynamics are unpredictable due to sensitivity to initial conditions, they are geometrically bound to a certain structure, the attractor. It is in this sense that chaos scientists are said to seek order in chaos.
______________________________________________________________________________________________
Reference
Devaney, Robert L. A First Course in Chaotic Dynamical Systems. New York: AddisonWesley, 1992.
Gleick, James. Chaos: Making a New Science. New York: Penguin Books, 1987.
Gulick, Denny. Encounters with Chaos. New York: McGraw-Hill, 1992.
Kellert, Stephen. In the Wake of Chaos. Chicago: University of Chicago Press, 1993.
Peitgen, Heinz-Otto, Hartmut Jurgens, and Dietmar Saupe. Chaos and Fractals: New Frontiers of Science. New York: Springer-Verlag, 1992.
Peitgen, Heinz-Otto, and Peter Richter. The Beauty of Fractals. Heidelberg: Springer Verlag, 1986.
Stewart, Ian. Does God Play Dice? The Mathematics of Chaos. Cambridge, MA: Black well Publishers, 1989.
الاكثر قراءة في نظريات ومبرهنات
اخر الاخبار
اخبار العتبة العباسية المقدسة
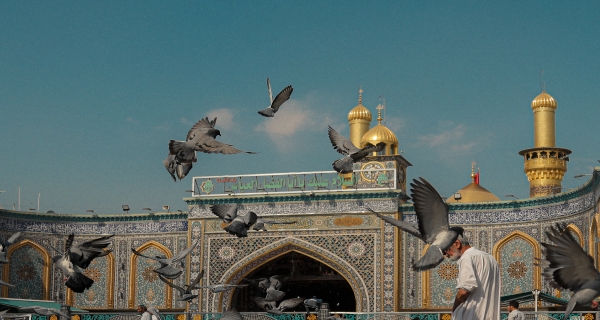
الآخبار الصحية
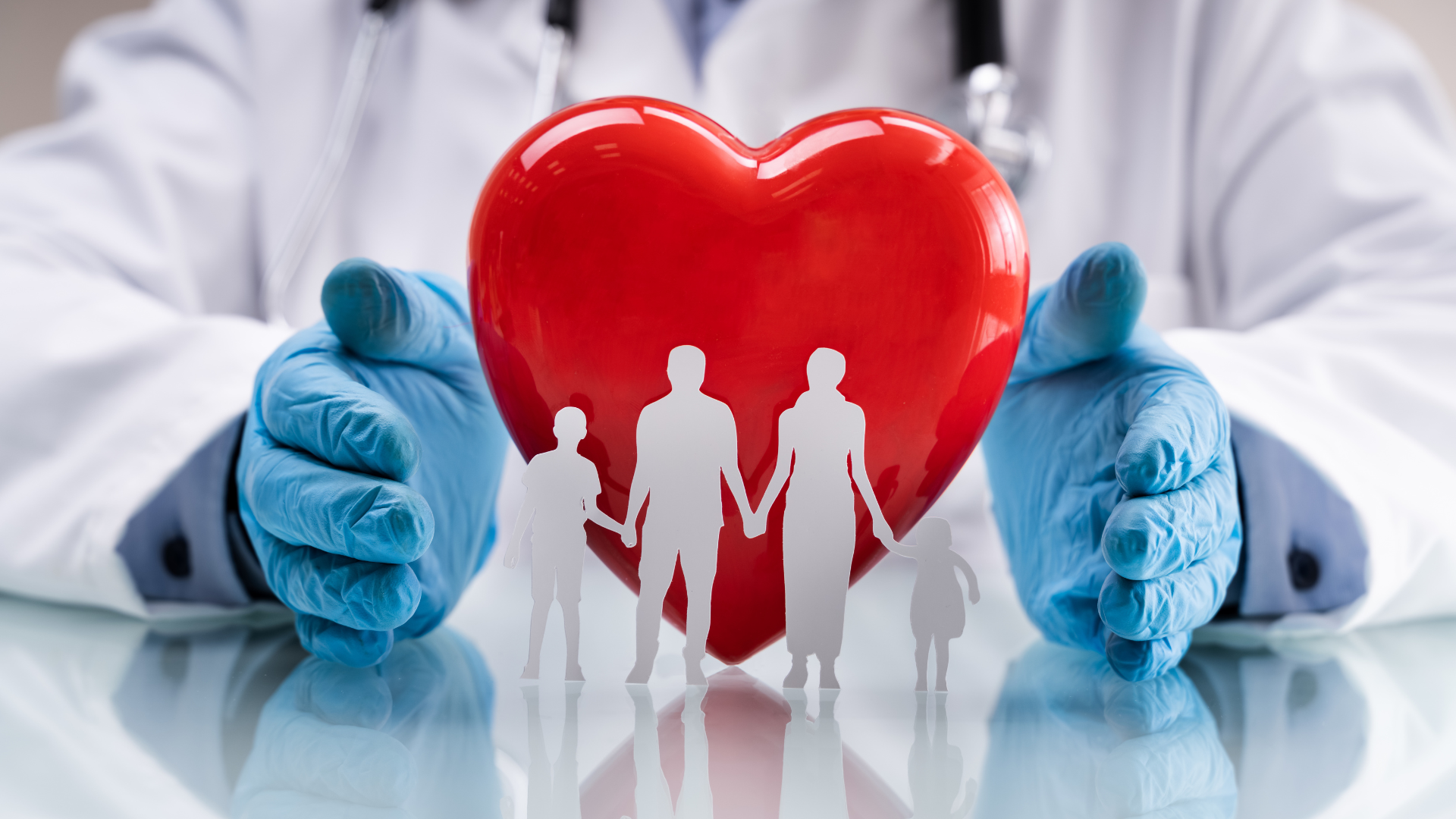